We Test Out A New Tool That Tells You What Your HDB Flat Is Worth: Does It Really Work?
-
Ryan J
- July 15, 2022
- 9 min read
- 2 2 Comments
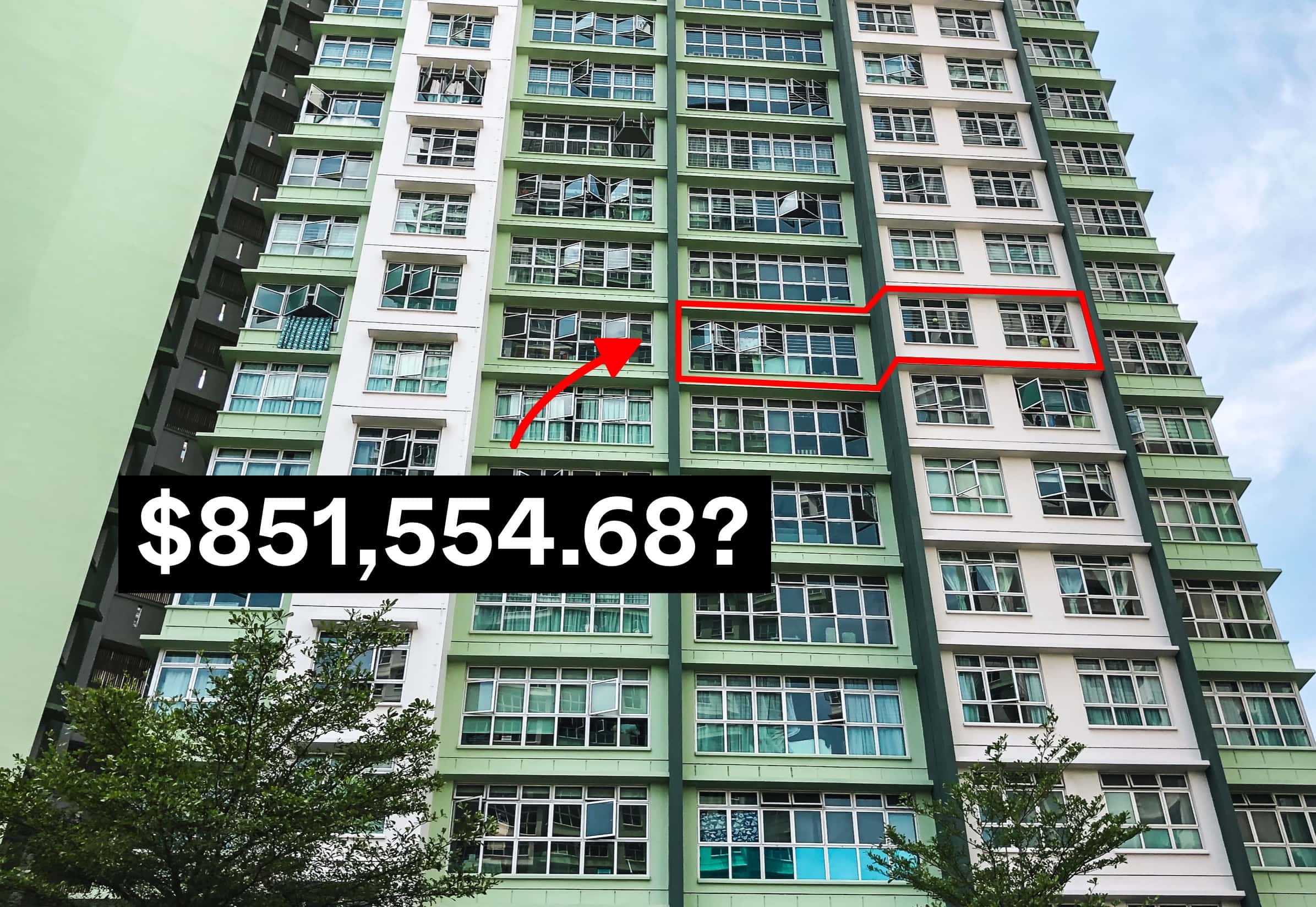
It’s the most common query anyone has when it comes to buying an HDB – how much is the flat worth?
Naturally, that’s a tough question to answer. While the majority of HDB flats do have the same layouts and features, there are still so many other factors to consider. Things such as location attributes, lease factors, views and facing, and even the condition of the flat can all play a major role in the price of an HDB.
And with the COV data not being published publicly like before, it can be hard to arrive at a relevant price sometimes.
This is why data scientists Michael McManus and Stuart Ong decided to build a pricing tool aptly named HDBestimate (you can see it in action here). You just have to input your address, flat type, floor level and size, and it will give you a price estimate, along with an “explanation” on how it arrived at that number. We spoke to them about how it works:
What is HDBestimate?
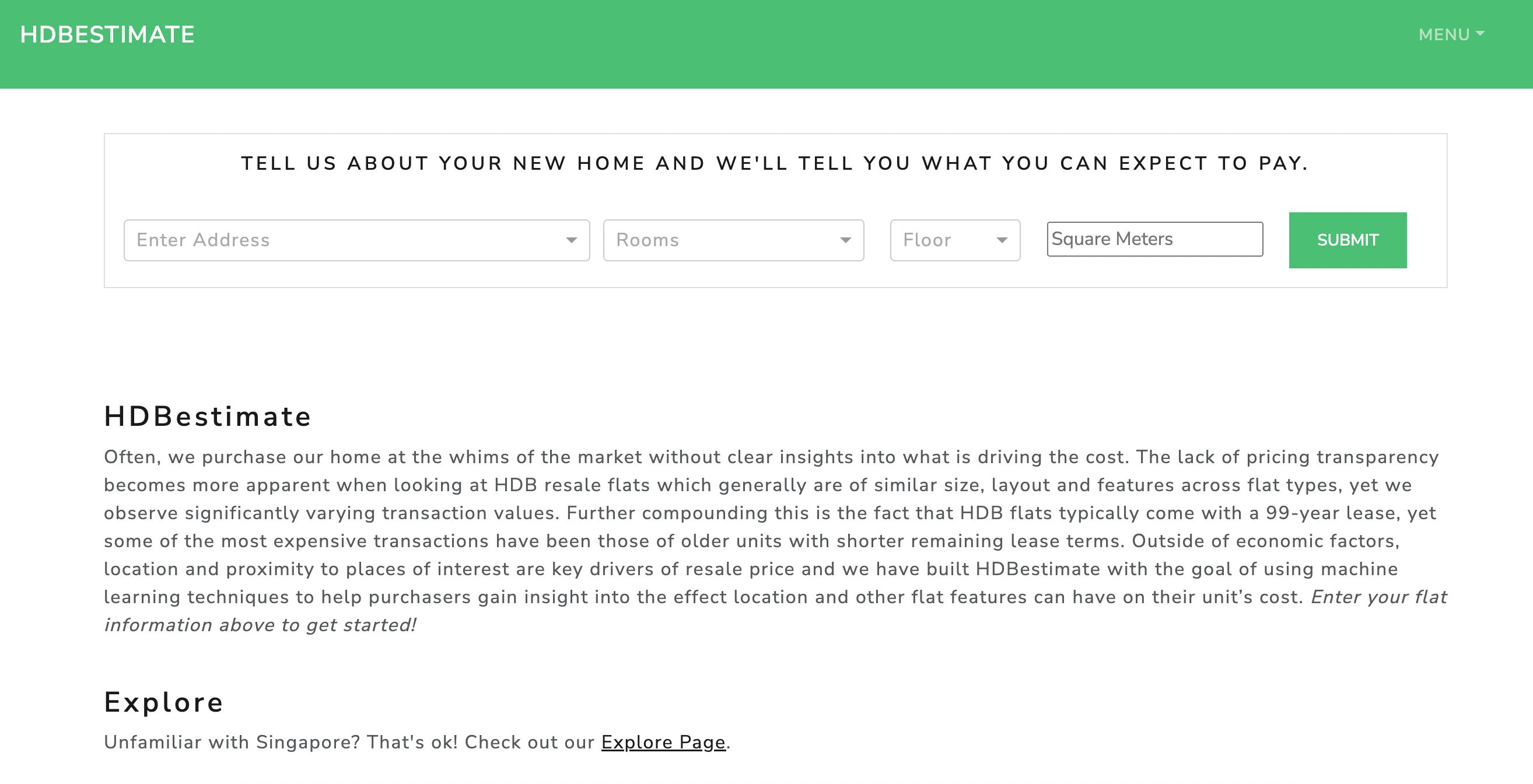
Right now, the method used to value HDB flats is mostly based on transaction history. When you ask the value, a property agent digs out URA transaction data, and checks what the other nearby flats sold for – it’s simple, but a bit one-dimensional.
HDBestimate uses an astounding 336 features, to estimate the value of your flat. The app also learns as it goes, and identifies the impact of various features on home prices.
“For every transaction, the model learns the relationship between the transaction price and the 336 features,” the developers explained, “So distance to a conservation area, like Tiong Bahru, is just one feature it is studying. After looking at all the transactions…the model essentially maps the relationship of each feature and its impact to price.”
That said, users won’t actually see all 336 features detailed, as that would just leave most of us with a headache. Rather, the app will highlight the 15 most relevant features of a flat’s price; that help you understand the most vital issues you face as a seller or buyer.
That’s the other great thing about HDBestimate: with many valuation tools, you don’t really understand how the numbers are derived. You get a valuation, but you don’t get to know why it might be higher or lower than surrounding units.
This was one of the issues Michael and Stuart wanted to solve:
“We felt it was not sufficient to just provide users with a model that estimated a price, but to also attempt to explain why the model was making certain predictions – and that is what the waterfall charts on the results page attempt to illustrate.”
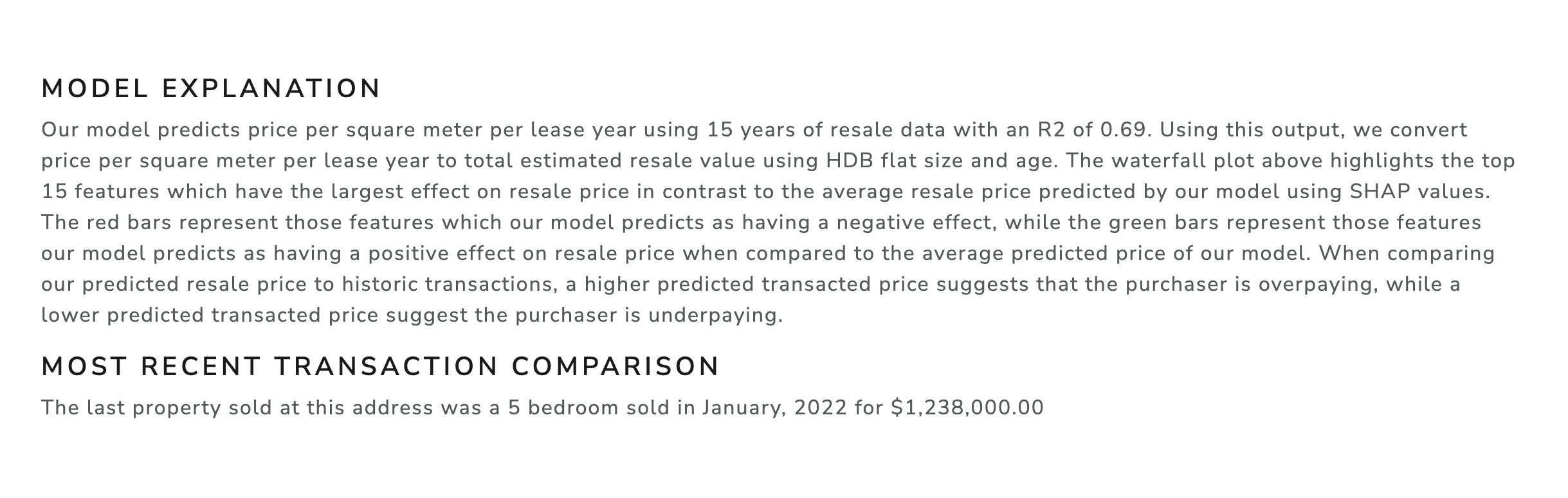
Why create such a tool?
When asked this, they answered simply, “while most are aware that location has a significant effect on real estate prices, how exactly location affects prices are less clear and that really was our key motivation in creating HDBestimate.”
To be clear, they wanted to help home buyers better understand and isolate the effects of the location versus a flat’s features or facilities that can typically add further complexity to an analysis of real estate prices.
Testing it out
Curious to see how this tool can help, we decided to put on our homebuyer hat to “hunt” for an HDB. For this exercise, we looked out for a 4-room flat in Tiong Bahru that’s close to the MRT. Here’s one we found:
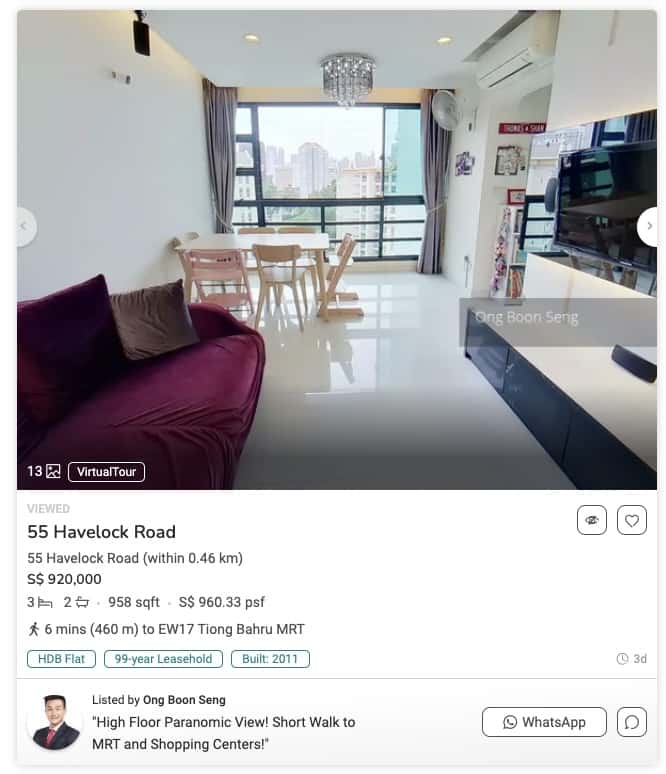
A 4-room flat for $920,000! Seems a little pricey, so we decided to check out the past transacted prices of this block from HDB’s website:
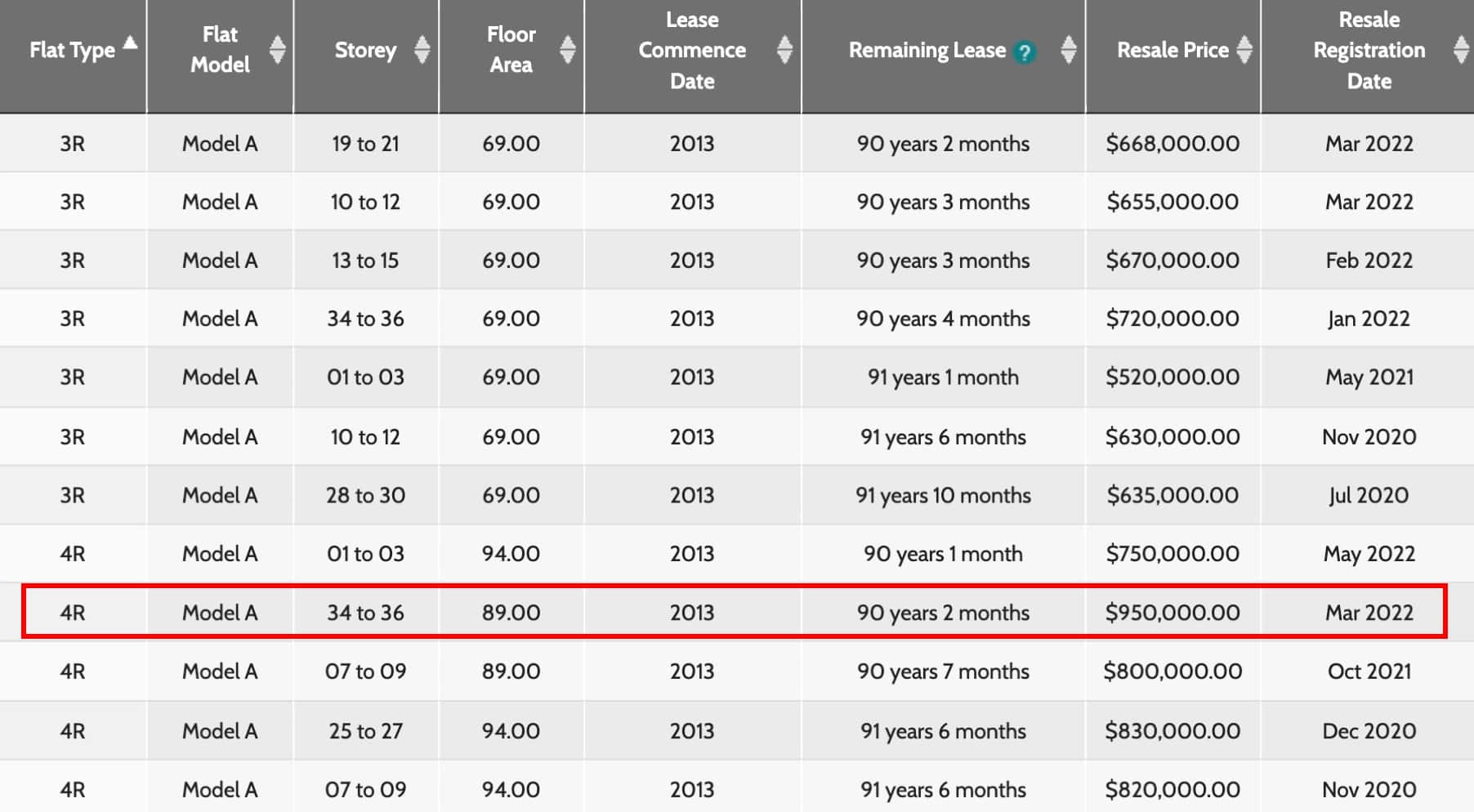
Considering a 34 to 36 floor unit went for $950K just 4 months ago, we can discount the floor premium from the price to derive an estimated price it can transact at.
Judging from the condominium opposite, this unit looks like it’s around the 15th floor. At around $5K per floor, an estimated 20 floors translate to a $100K difference between both units. Perhaps this unit should go for $850K instead?
We put in the information and hit “submit”!

After loading for around 5 seconds, the result page shows up with the estimated pricing of $854,619.37.
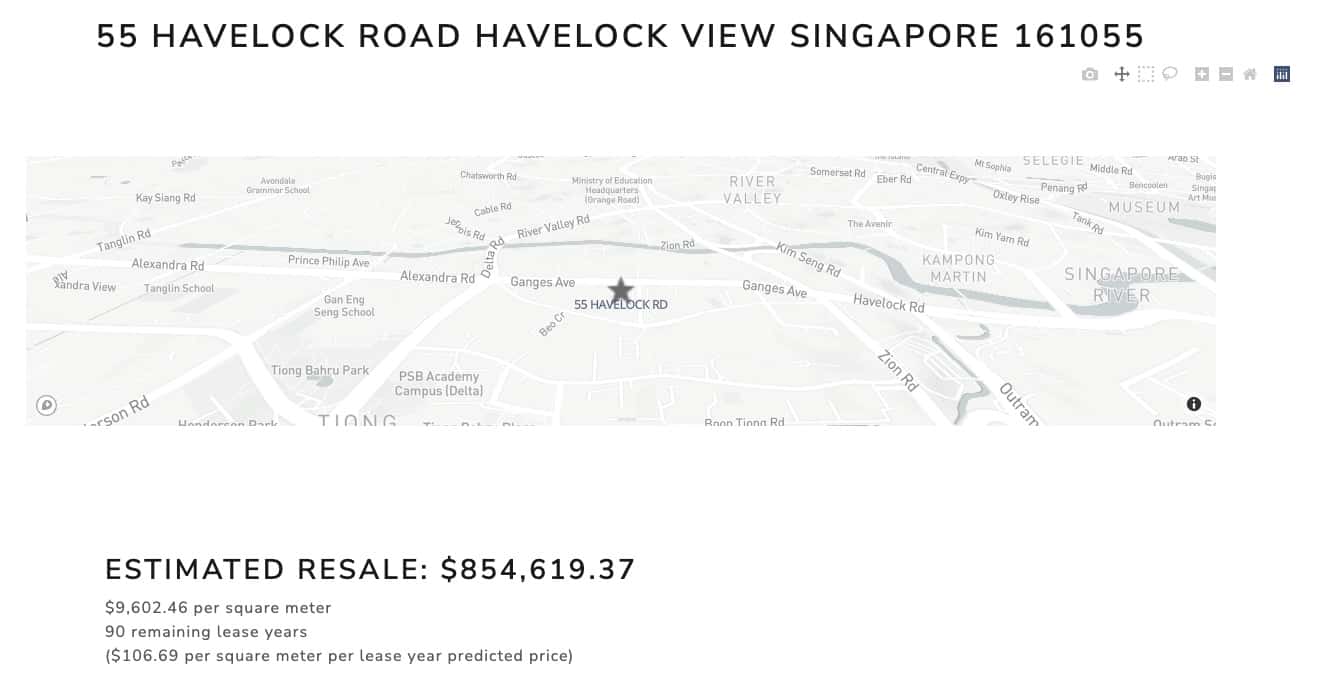
Hmm, an estimated $854K – this is a lot closer to what we would’ve estimated. The seller here seems to be asking for around 7.6% above the estimated valuation from the tool!
Below that, you can also see a graph showcasing the factors taken into consideration and how they affect the outcome:
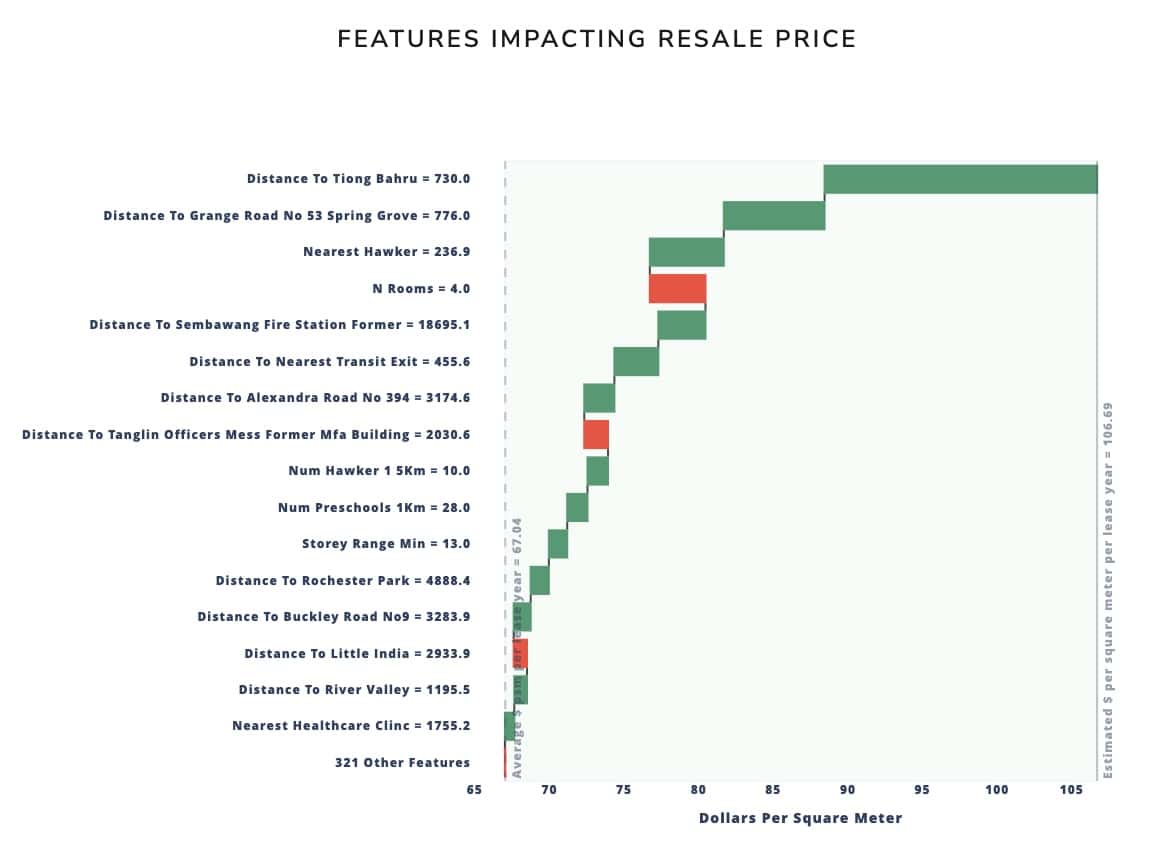
How do you read the graph?
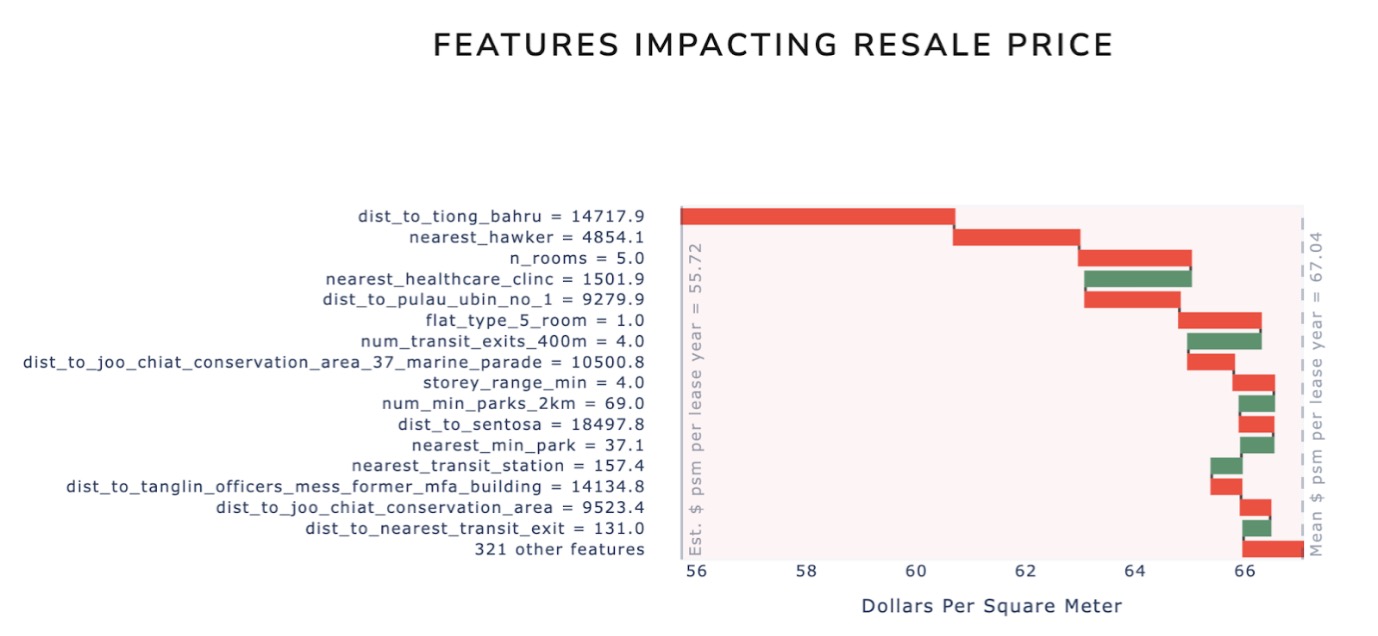
Red indicates a negative effect (lowering the price versus the average), while green indicates a positive effect (raising the price versus the average).
“So in the screenshot here, this property is around 14.7km away from Tiong Bahru (the Y axis) which has a $3 to $4 negative effect on price (see the X-axis and red bar), versus the average prediction of the model,” they explained.
“With the average predicted price of $67.04 as the starting point, the feature effects are added or subtracted accordingly, which results in the final predicted price of $55.72 for this particular property.”
Why use so many features to determine the price?
Michael and Stuart said that: “We do caution that while it is tempting to link a predicted price to one or a few key features, our model makes a prediction based on all 336 features – and is not reliant on any one single feature.”
While we don’t know the full list of 336 features, they did let us in on some of them:
“Beyond the usual features like the number of MRT/LRT stations or schools within a specific radius, we used conservation areas as a proxy for areas of interest, which turned out to be representative of desirable locations.
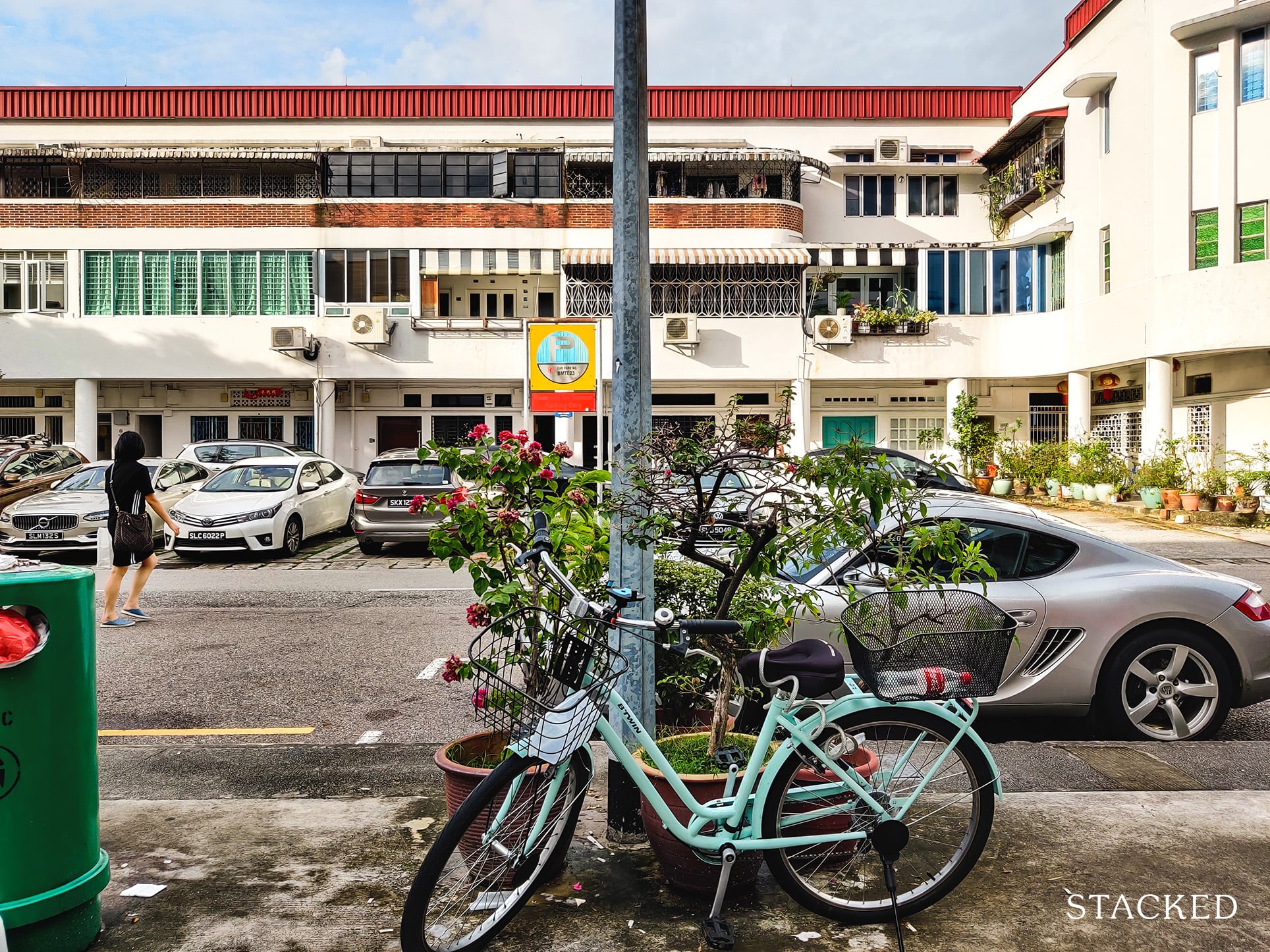
These features helped encapsulate popular areas like Tiong Bahru, Holland Village or Dempsey Hill, as well as other important areas like the CBD. which resulted in the model awarding some premium or discount, depending on how far the location was to these areas.”
And in case you’re wondering, yes, the age of the flat is also accounted for:
“Our model is trained on price per square foot, per remaining lease year, where each transaction is normalised for age. This is also the output of our model. and when an address is entered. we check for the remaining lease year of the blocks to get the final valuation.”
As with human valuation experts though, HDBestimate doesn’t yet account for the effects of renovation.
Michael and Stuart explained: “Our tool does not explicitly account for flat-specific features beyond flat type or the number of rooms, such as renovation. Such features are generally quite uniform across the different types of flats.
Location features were our key focus when we set out to build this. and this is definitely a limitation of both the model. as well as the data – as that information for each transaction is not readily available. We believe that our model does a good job of generalising the price of the average unit. but will not be able to account for unique flat-specific features that some may believe might garner a premium.”
To be fair, no one has ever found an accurate way to determine what renovations contribute. What’s gorgeous to one owner is hideous to another; and there is, as the saying goes, no accounting for taste.
HDBestimate has already made some interesting observations
“Interestingly, we are seeing that 4-room flats generally result in a premium over 5-room flats,” they told us.
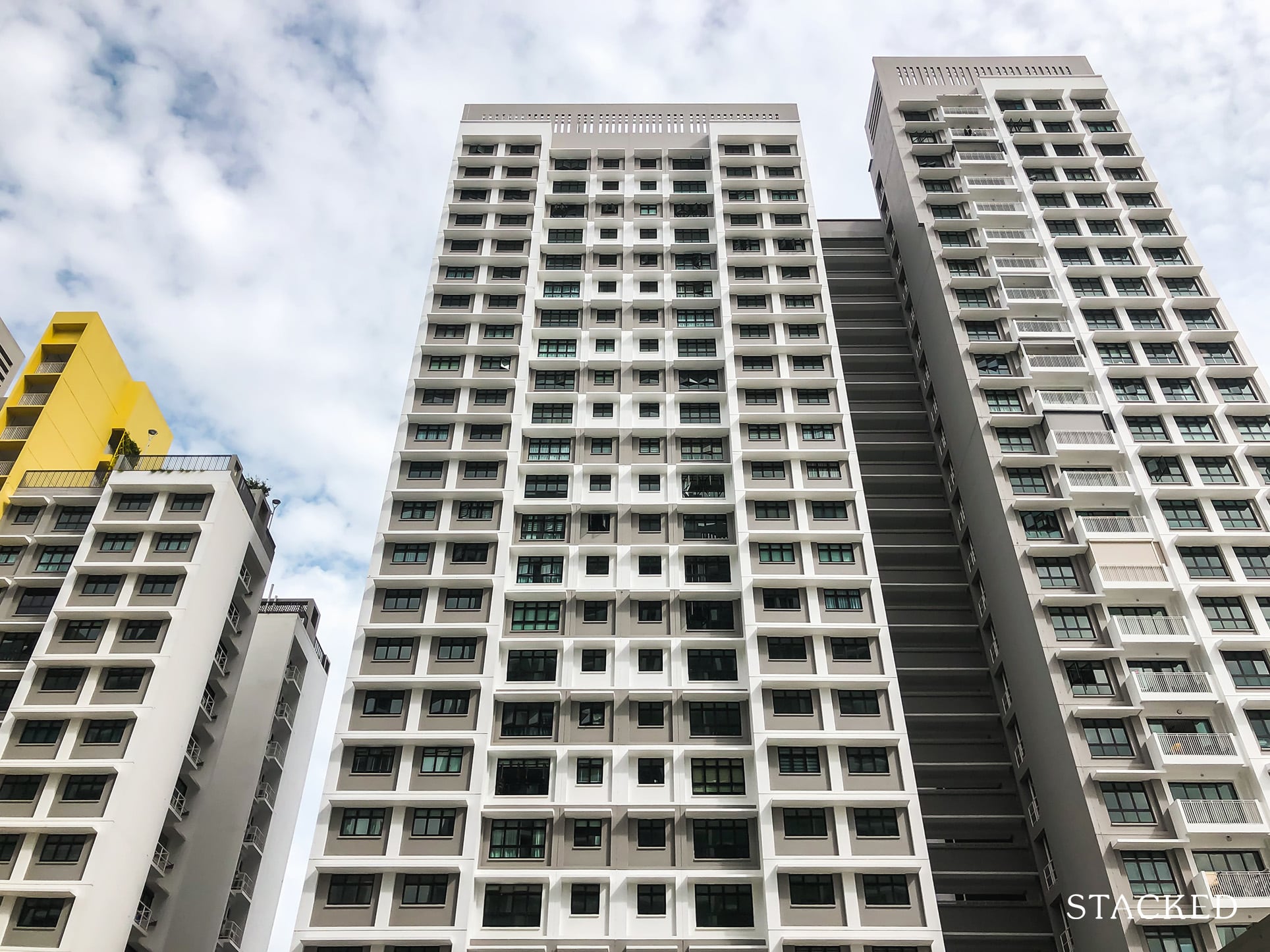
“At this point, we aren’t entirely sure if larger flats are harder to sell. and hence have to be priced cheaper; or if what we’re seeing is a quirk of the data, where some of the more expensive transactions in recent years have been the older units that happen to be 4-room flats. This is one of the things that we are looking to explore further.”
That would run contrary to the common belief that larger homes are always more desirable; and figuring out why would be helpful to the property industry as a whole.
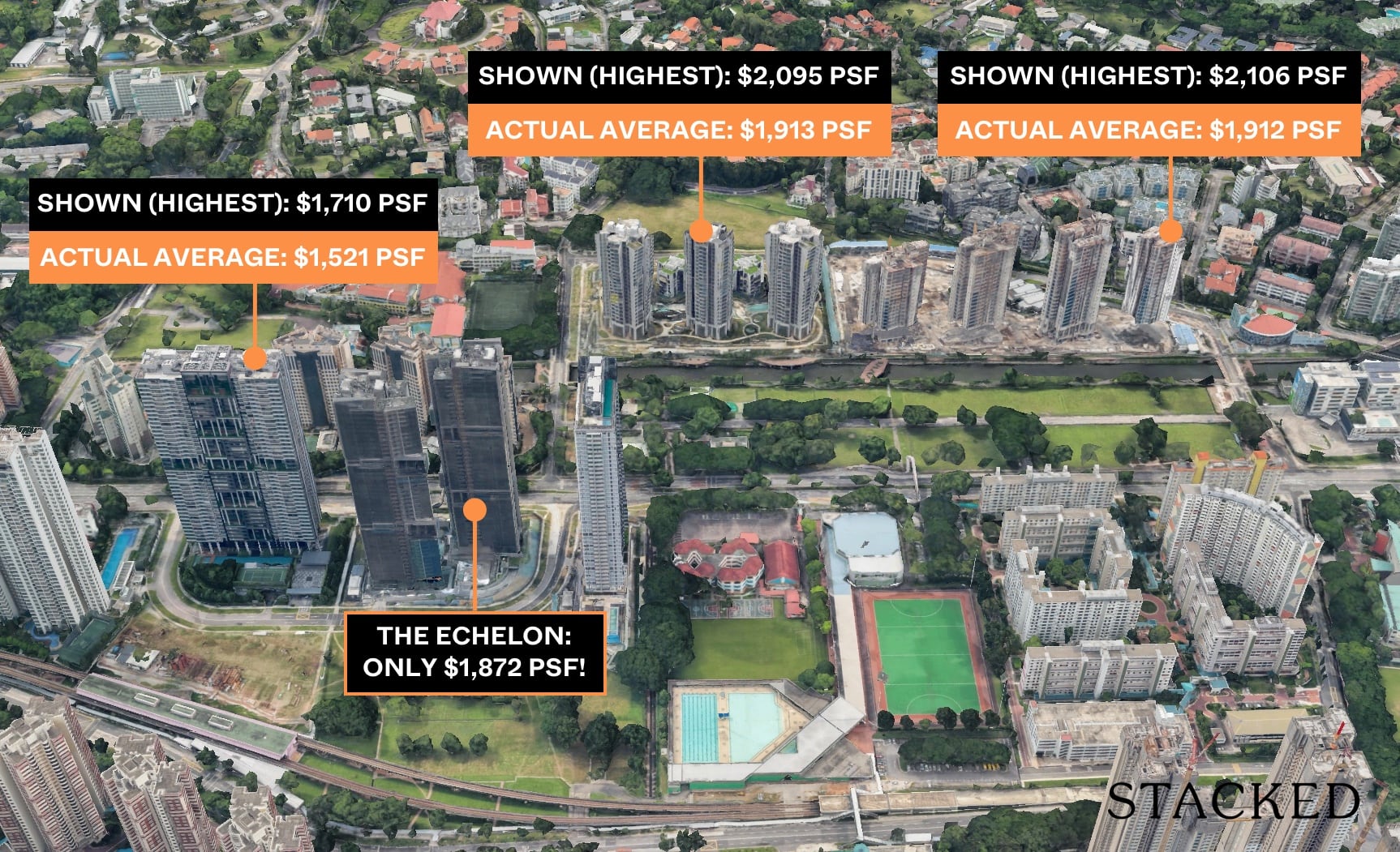
Buying7 Real Life Examples Of How Property Data Can Be Used To Mislead Home Buyers
by Ryan JHow should we use HDBestimate once it’s out?
HDBestimate provides an additional point of reference when you’re trying to determine the price of a flat. This is a figure that would go beyond price data, which isn’t always available or accurate.
They added: “We hope that this can help augment the decision-making process, by enabling users to make more informed decisions.
For instance, our model might predict a high premium-to-price for being close to a specific location or feature; but the user might not value that specific feature or location as much, and could find alternative locations as a result, or at least have a clearer idea of what they are paying for.”
For example, HDBestimate might point out that you’re paying a lot more for a given HDB flat, because it’s close to a train station that includes multiple MRT lines. However, if you drive rather than use the train, you might decide it’s not worth paying such a high premium for that feature.
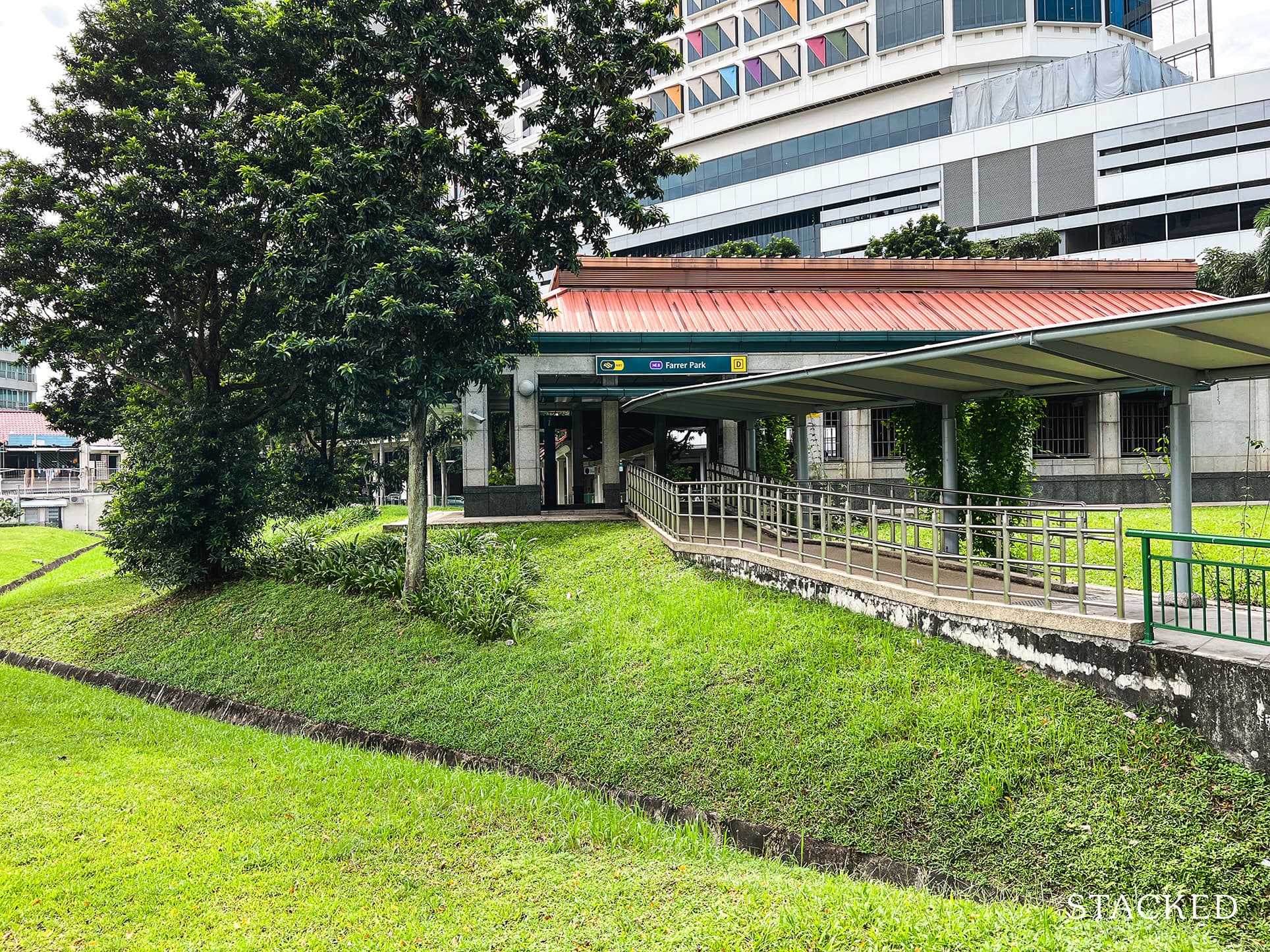
While Michael and Stuart didn’t explicitly say so, we think this could also be of use to property developers, and perhaps HDB itself. If we can better understand the factors that drive prices, we can build homes in ways that are truly important to buyers.
How accurate is HDBestimate right now?
Michael and Stuart told us that “Our current model predicts with an R^2 of 69%, which we believe isn’t too bad for real-world data that encompasses human emotions, as well as actions that are far less predictable.”
There are some invariable challenges to be faced here – such as the possibility that people have been paying more over the last couple of years. (Resale flat prices went on an upswing just after Covid-19, after almost eight years of consecutive decline).
“The model could have some trouble predicting premiums on things it has never seen people pay premiums for,” the app developers say, “We have some additional ideas on how we can potentially further improve the accuracy and account for issues such as overfitting in future iterations.”
For those who are interested to try the new tool out, simply click the link here.
About the creators:
Michael McManus and Stuart Ong just completed their Masters of Applied Data Science, from the University of Michigan. Michael works as a senior data analyst for a utility company in the United States, while Stuart works as a portfolio manager for a private bank in Singapore.
HDBestimate has been the product of several months of work, and is part of their capstone project for the pair’s Masters program. It is a work in progress.
For more on this as it unfolds, follow us on Stacked. We’ll also provide you with in-depth reviews of new and resale properties alike, and news on the latest happenings in the Singapore property market.
Diving deeper into the model
For those who are curious about the inner workings of the models, here are some insights they’ve kindly shared with us!
1) What were the challenges you faced while building this?
Feature engineering location features were a big part of this project and came with a host of challenges in terms of not only collecting the location data but then geospatially connecting the various location features to each transaction.
The time element also created some challenges for us as we had to adjust all prices for inflation so that the model can make predictions based on prices today. In addition, while there was a time element to prices, there was no time element to location features as this information isn’t readily available.
To overcome this, we reduced our period of analysis to 12 years of data instead of the full 30 years of transactions as we felt that most of the features were substantial in nature (hospitals and MRT stations vs the location of something that is easily moved).
2) What is meant by “feature engineering location features”?
The model uses location features to estimate the price and these features are not readily available so we had to engineer them.
For instance, for MRT stations we had to first locate all the MRT stations and then create a score based on the number of lines it connects to as a connectivity score. Then for each transaction, we would sum the connectivity scores of the stations in a 400m radius which would then become a location feature we would then train our models on.
3) How does the model determine the extent to which a property is affected by these features?
After looking at all the transactions in the training data, the model essentially creates a map of the relationship of each feature and its impact on the price (simplistically you can think of it as linear regression equations and the effects are the coefficients).
What we do when you search an address is get the 336 features and pass that to the model which then estimates the price based on the values.
4 How do you determine if the 336 features are relevant to the estimated valuation of the HDB?
Ultimately, if the model determines the feature is not relevant it will weigh its effect as zero (or close to it) – this happens during the training of the model.
This link here aint working on mobile or desktop devices – https://hdb-estimate.herokuapp.com/https://hdb-estimate.herokuapp.com/
Hmm it does work for us though! Could you try https://hdb-estimate.herokuapp.com/ ?